Adjusting to Automation
Policymakers are understandably concerned about the effect of automation technologies on employment and wage levels in their jurisdictions. The effects, however, tend to be quite heterogeneous – and not much is known regarding what drives this heterogeneity. This makes devising the right policies a bit tricky.
Luckily, a Pillars team made up of Fabien Petit and his colleagues Tommaso Ciarli and Florencia Jaccoud is focusing their research on this issue.
In their latest paper, they report on the findings of a study they conducted on the labour market adjustments brought about in 227 regions across 22 European countries by four automation technologies, namely robots, communication technology, information technology and what they label software/databases, from 1995 to 2017.
To find the roots for the heterogeneous responses to technology penetration, they first clustered the regions on the basis of their sectoral specialisation and technology capabilities. This gifted them the insight that labour market adjustments to automation differ along precisely those features. In this respect, their analysis unearthed a huge divide between Southern and Eastern, and Northern and Western European regions, and between service-intensive and industry/agriculture-intensive regions.
The adjustments also depend on the technology in question: for all the hype about robots, they found that their introduction actually has a positive effect on employment, whereas in the case of IT, CT and software, the implications vary depending on their degree of penetration through the industry or services sectors.
At any rate, part of the response to automation technologies is substantial employment reallocation among sectors, mostly concerning low-paid workers. Therefore, local public policies aimed at introducing such technologies should consider two key factors: first, local specificities such as regional workforce specialization and technology capabilities and, second, given that reallocated workers tend to be those at the bottom of the wage distribution, suggesting that they are the least skilled, the provision of training to ensure that these reallocated workers continue to be employable.
The public sector also plays a role. It is affected by changes in technology penetration in its own and other sectors, and is often the recipient of displaced workers. Therefore, the role of the public-service sector in employment should be carefully considered when assessing the consequences of automation on employment.
Given that the authors focus on sectoral employment, they would welcome the availability of more and better data on occupations and skills to provide a more thorough understanding of worker reallocation. Luckily, work by another Pillars’ team has just created a perfect tool for this.
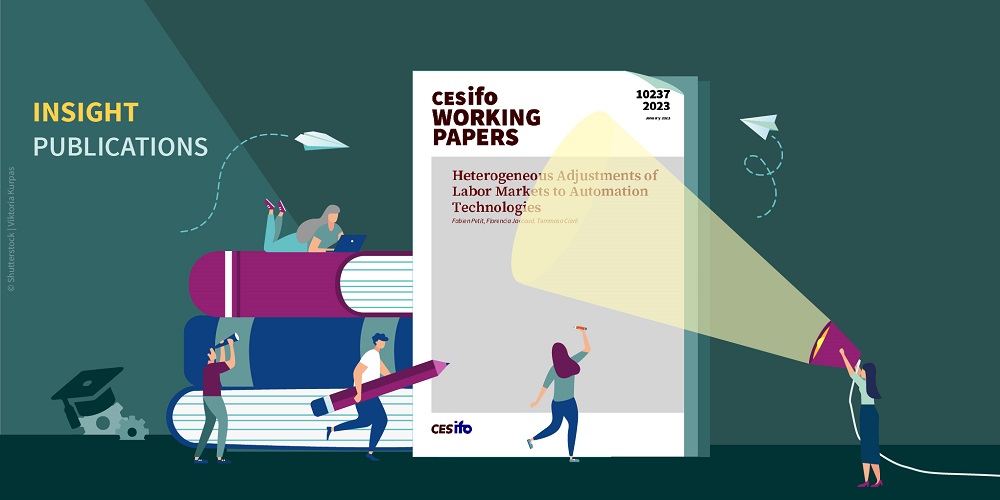